Research
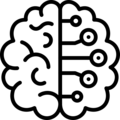
Machine Learning Methods
The group is interested in understanding and developing machine learning models applicable to challenges posed by analysing biomedical data. With a long history in developing large-scale learning methods for sequence classification on genomes, and experience in clinical data analysis, the group seeks to advance state of the art in deep learning, probabilistic modelling, and time-series analysis. [Read more ...]
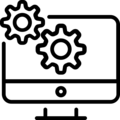
Machine Learning Applications
The group develops innovative methods for the analysis of electronic health records (EHR) with the objective of automatically summarising patient states over time and doing predictive modelling. Ultimately, computational models of patient state can aid in decision support systems using EHR, integrating genomic information and other data sources to provide comprehensive, automated suggestions for treatments, prognoses, and to assist in clinical trial enrolment. [Read more ...]
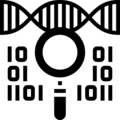
Sequence Analysis in the Biomedical Domain
The availability of fast and affordable high-throughput DNA and RNA sequencing have transformed biology and medicine into research areas of data science. We are working on a compressed, distributed storage system for reference genomes and DNA sequencing data that dynamically scales to the various needs of individual research projects. [Read more ...]
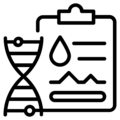
Computational Multi-Omics
The Biomedical Informatics Lab has been an active contributor to the analysis of biomedical *omics data for over 15 years. During this time, we have gained a rich experience in scalable data analysis and methods development, starting with the analysis of whole-genome microarray data, over transcriptome tiling arrays, exome, genome, and transcriptome sequencing data, to metagenomic data analysis. Over the recent past, we have expanded our research to include additional data modalities, such as single-cell profiling, proteomics, and metabolomics data. Our work in this area ranges from methods development over the experimental design to data integration and applied machine learning. [Read more ...]
The list of (most) lab publications is available here and the Google Scholar profile of Gunnar Rätsch is available here.